Stunning Info About How To Check Linearity Of Data Basic Line Chart
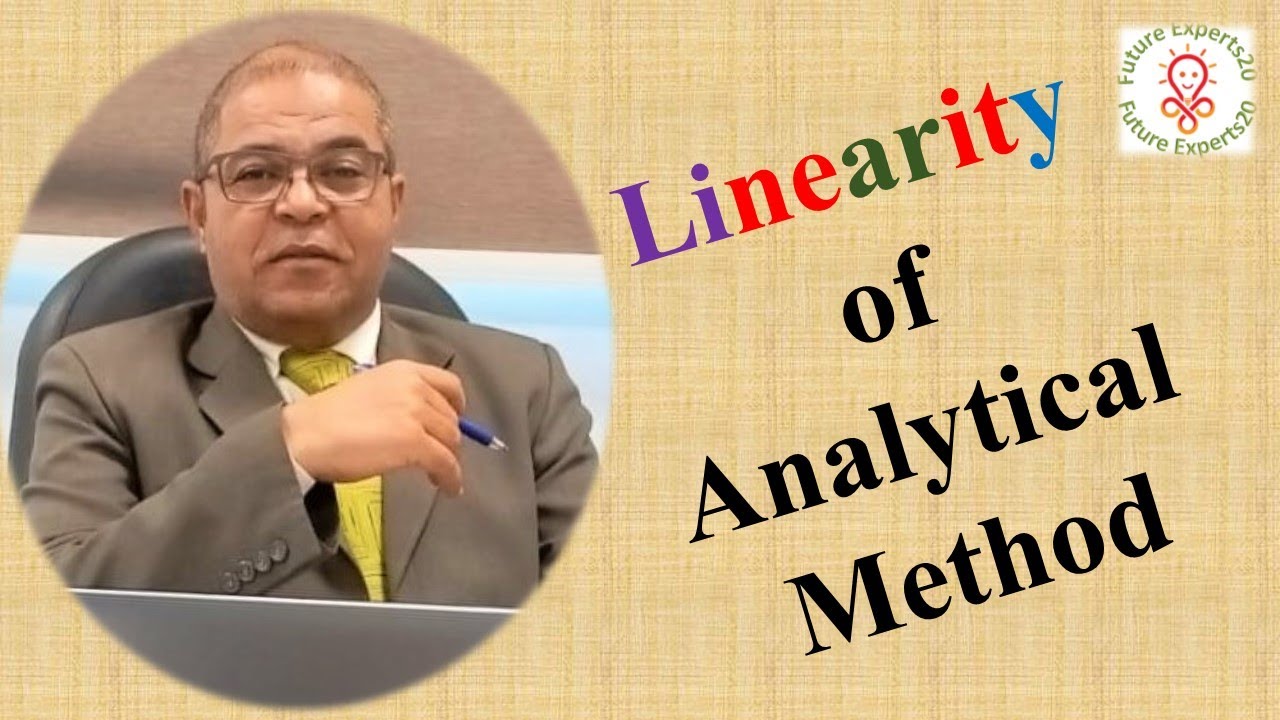
A linear regression model assumes that the average outcome is linearly related to each term in the model when holding all others fixed.
How to check linearity of data. For simplicity, i only take the numeric variables. To check this assumption, it’s pretty easy. These assumptions are a vital part of assessing whether the model is correctly specified.
A simple pairplot of the dataframe can help us see if the independent variables exhibit linear relationship with. This can be done in two ways: The model used a continuous response variable and categorical explanatory variables.
I have used simple linear regression, and i'm now checking that the model meets the assumption of linearity. How to test the linearity assumption using python. Checking the 1st assumption:
The presence of a pattern may indicate a problem with some aspect of the linear model. In this short tutorial, we will see how we can tell if the data is linear or not. That’s why i drop the only boolean feature — chas.
For our calculations, the following sample (x, y) pairs will be used: That is, the red line should be approximately horizontal at zero. The equally spread residuals around a horizontal line without distinct patterns are a good indication of having the linear relationships.
The linearity test is one of the assumption tests in linear regression using the ordinary least square (ols) method. Use the residual plots to check the linearity and homoscedasticity. So, among others i check the linear dependency between my dependent (which is continuous) and my independent (nominal or dummy) variables.
The objective of the linearity test is to determine whether the distribution of the data of the dependent variable and the independent variable forms a linear line pattern or not? Create a scatter plot with x and y. For this article, i use a classic regression dataset — boston house prices.
2.4, 3.4, 4.6, 3.7, 2.2, 3.3, 4.0, 2.1. The dependent variable and independent variable must have a linear relationship. In a model (y = b0 + b1.x + etc.), i want to know whether it is acceptable to assume linearity for (x).
Linearity between the x and y. If the plot shows a distinct trend, you can conclude that there is some amount of linearity between the two variables. Linearity is a quantitative assessment of how strongly related a set of data is.
The svd breaks data up into component pieces ranked by magnitude of their contribution to the whole. However, if the assumptions are not satisfied, the interpretation of the results will not always be valid. At the moment i am trying to make a list of the different approaches that could be used to verify the linearity of an effect.