Impressive Tips About Which Is The Simplest Time Series Forecasting Model Trendline Power
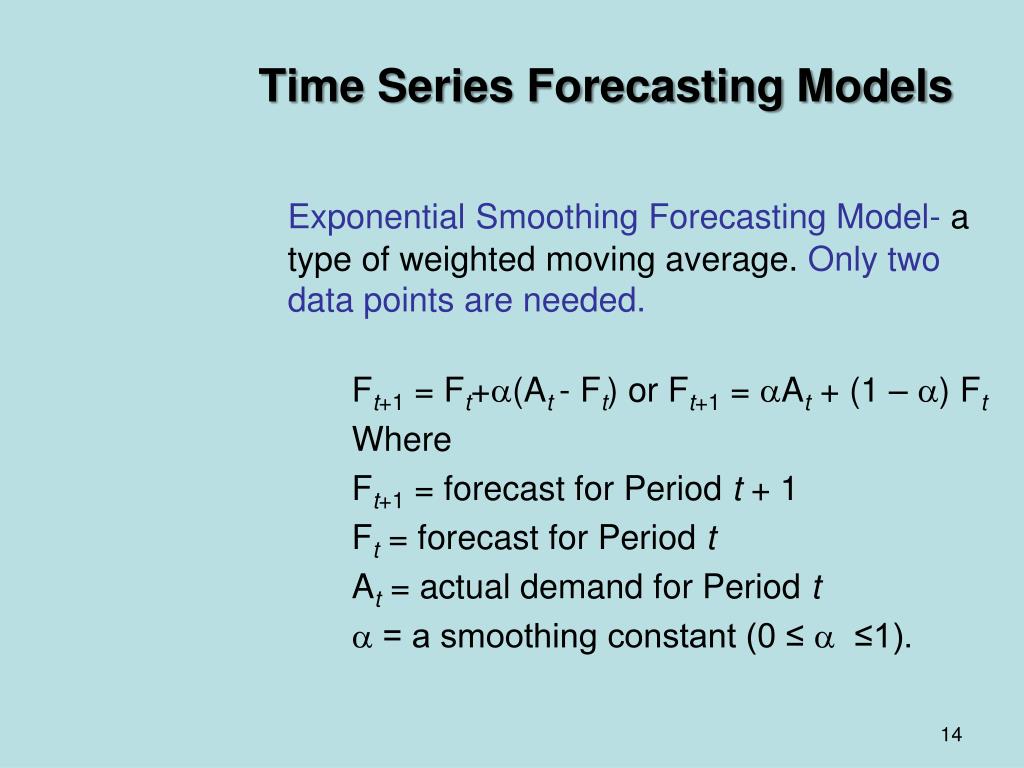
Examples across industries include forecasting of weather, sales numbers and stock prices.
Which is the simplest time series forecasting model. This cheat sheet demonstrates 11 different classical time series forecasting methods; Such a shift might happen in the future to deep learning models. We describe 10 forecasting models and we apply them to predict the evolution of an industrial production index.
In statistical terms, time series forecasting is the process of analyzing the time series data using statistics and modeling to make predictions and informed strategic decisions. Photo by drew beamer on unsplash. How to automate the expanding window model.
A method that requires little or no training or intelligence. Notes on the random walk model. However, this might change in the future.
If you’re a business owner and have ever wanted to predict the future, then you’re in luck. Time series forecasting essentially allows businesses to predict future outcomes by analyzing previous data, and providing businesses with a glimpse into what direction data are trending. We saw a shift from statistical models, which dominated time series forecasting for a long time, to ml models.
The complete guide to time series models. Introduction to forecasting: Using arima model, you can forecast a time series using the series past values.
The statistical tools that assist in forecasting a time series are called the time series forecasting models. An overview of time series forecasting models. Matthew urwin | aug 01, 2023.
The three types of forecasting. Time series forecasting is the task of predicting future values based on historical data. A method that is deterministic, meaning that it produces an expected output given the same input.
Now that we understand that time series might contain different components and that there are approaches for their decomposition, we can introduce several simple forecasting methods that can be used in practice, at least as benchmarks. A method that is fast to implement and computationally trivial to make a prediction. Their usage aligns with the idea of forecasting principles discussed in section 1.2.
You will also see how to build autoarima models in python. How to automate the persistence model and test a suite of persisted values. A time series model is a set of data points ordered in time, and it’s used in forecasting the future.
The time series forecasting models can be broadly classified into simple models (mean model, linear trend model, random walk model) , average and smoothing models (moving average, exponential smoothing) , linear regression models ,. How to automate the rolling window forecast model and test a suite of window sizes. Time series forecasting involves analyzing data that evolves over some period of time and then utilizing statistical models to make predictions about future patterns and trends.